3D model reconstruction using neural gas accelerated on GPU |
| |
Affiliation: | 1. State Key Laboratory of Coastal and Offshore Engineering, Dalian University of Technology, Dalian 116024, China;2. School of Information Engineering, Dalian Ocean University, Dalian 116023, China |
| |
Abstract: | 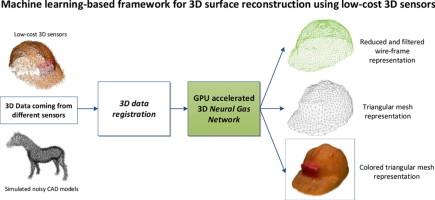 In this work, we propose the use of the neural gas (NG), a neural network that uses an unsupervised Competitive Hebbian Learning (CHL) rule, to develop a reverse engineering process. This is a simple and accurate method to reconstruct objects from point clouds obtained from multiple overlapping views using low-cost sensors. In contrast to other methods that may need several stages that include downsampling, noise filtering and many other tasks, the NG automatically obtains the 3D model of the scanned objects. To demonstrate the validity of our proposal we tested our method with several models and performed a study of the neural network parameterization computing the quality of representation and also comparing results with other neural methods like growing neural gas and Kohonen maps or classical methods like Voxel Grid. We also reconstructed models acquired by low cost sensors that can be used in virtual and augmented reality environments for redesign or manipulation purposes. Since the NG algorithm has a strong computational cost we propose its acceleration. We have redesigned and implemented the NG learning algorithm to fit it onto Graphics Processing Units using CUDA. A speed-up of 180× faster is obtained compared to the sequential CPU version. |
| |
Keywords: | Neural gas Topology preservation 3D model reconstruction Graphics Processing Units |
本文献已被 ScienceDirect 等数据库收录! |
|