基于自适应LASSO先验的稀疏贝叶斯学习算法 |
| |
引用本文: | 白宗龙, 师黎明, 孙金玮. 基于自适应LASSO先验的稀疏贝叶斯学习算法. 自动化学报, 2022, 48(5): 1193−1208 doi: 10.16383/j.aas.c210022 |
| |
作者姓名: | 白宗龙 师黎明 孙金玮 |
| |
作者单位: | 1.哈尔滨工业大学仪器科学与工程学院 哈尔滨 150006 中国;;2.奥尔堡大学建筑设计与媒体艺术系 奥尔堡 9000 丹麦 |
| |
基金项目: | 中央高校基本科研业务费项目(IR2021222)资助~~; |
| |
摘 要: | 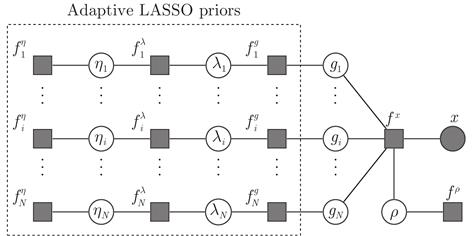 为了提高稀疏信号恢复的准确性, 开展了基于自适应套索算子(Least absolute shrinkage and selection operator, LASSO)先验的稀疏贝叶斯学习(Sparse Bayesian learning, SBL)算法研究. 1) 在稀疏贝叶斯模型构建阶段, 构造了一种新的多层贝叶斯框架, 赋予信号中元素独立的LASSO先验. 该先验比现有稀疏先验更有效地鼓励稀疏并且该模型中所有参数更新存在闭合解. 然后在该多层贝叶斯框架的基础上提出了一种基于自适应LASSO先验的SBL算法. 2) 为降低提出的算法的计算复杂度, 在贝叶斯推断阶段利用空间轮换变元方法对提出的算法进行改进, 避免了矩阵求逆运算, 使参数更新快速高效, 从而提出了一种基于自适应LASSO先验的快速SBL算法. 本文提出的算法的稀疏恢复性能通过实验进行了验证, 分别针对不同大小测量矩阵的稀疏信号恢复以及单快拍波达方向(Direction of arrival, DOA)估计开展了实验. 实验结果表明: 提出基于自适应LASSO先验的SBL算法比现有算法具有更高的稀疏恢复准确度; 提出的快速算法的准确度略低于提出的基于自适应LASSO先验的SBL算法, 但计算复杂度明显降低.

|
关 键 词: | 稀疏信号恢复 稀疏贝叶斯学习 自适应LASSO先验 贝叶斯推断 |
收稿时间: | 2021-01-12 |
|
| 点击此处可从《自动化学报》浏览原始摘要信息 |
|
点击此处可从《自动化学报》下载免费的PDF全文 |
|