基于单通道多尺度图神经网络的自动调制识别 |
| |
引用本文: | 国强, 聂孟允, 戚连刚, Kaliuzhnyi Mykola. 基于单通道多尺度图神经网络的自动调制识别[J]. 电子与信息学报, 2023, 45(5): 1575-1584. doi: 10.11999/JEIT220840 |
| |
作者姓名: | 国强 聂孟允 戚连刚 Kaliuzhnyi Mykola |
| |
作者单位: | 1.哈尔滨工程大学信息与通信工程学院 哈尔滨 150001;;2.先进船舶通信与信息技术工业和信息化部重点实验室 哈尔滨 150001;;3.哈尔科夫国立无线电电子大学 哈尔科夫 61166 |
| |
基金项目: | 国家重点研发计划(2018YFE0206500),国家自然科学基金(62071140),中央高校基本科研业务费专项资金(3072022QBZ0801) |
| |
摘 要: |  针对自适应可见性图(AVG)算法复杂度过高且精度提升不明显的缺点,该文提出一种基于单通道多尺度图神经网络(SMGNN)的自动调制识别(AMR)框架,并对框架各个部分进行了可解释性研究。首先利用多层感知机和1维卷积自适应地实现了单通道信号序列和图之间的映射,有效降低了AVG算法的复杂度;其次,设计了一种多尺度图神经网络,将不同分辨率的特征进行融合,提升了模型识别准确率。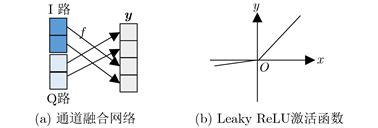 实验表明,该文提出的SMGNN算法相比于AVG算法节省了近1/2的参数量,且识别精度得到了较大的提升。
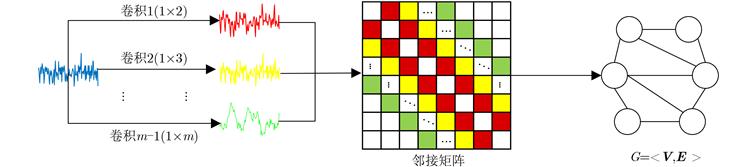
|
关 键 词: | 自动调制识别 图神经网络 自适应可见性图 特征融合 |
收稿时间: | 2022-06-24 |
修稿时间: | 2022-11-08 |
|
| 点击此处可从《电子与信息学报》浏览原始摘要信息 |
|
点击此处可从《电子与信息学报》下载免费的PDF全文 |
|