基于强化学习的减少烘丝过程中烟丝 “干头” 量的方法 |
| |
引用本文: | 毕素环, 蒋一翔, 于树松, 丁香乾, 牟亮亮, 王彬. 基于强化学习的减少烘丝过程中烟丝 “干头” 量的方法. 自动化学报, 2023, 49(8): 1679−1687 doi: 10.16383/j.aas.c190367 |
| |
作者姓名: | 毕素环 蒋一翔 于树松 丁香乾 牟亮亮 王彬 |
| |
作者单位: | 1.中国海洋大学信息科学与工程学院 青岛 266000;2.青岛理工大学信息与控制工程学院 青岛 266520;3.浙江中烟工业有限责任公司 杭州 310000;4.中国海洋大学继续教育学院 青岛 266000 |
| |
基金项目: | 国家重点研发计划(2017YFA0700601)资助~~; |
| |
摘 要: | 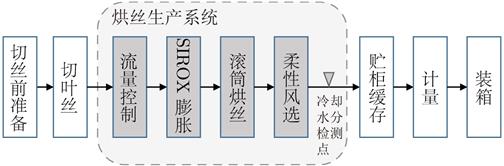 针对烘丝开始阶段存在的烘丝温度超调、过干烟丝较多等问题, 提出一种基于强化学习 (Reinforcement learning, RL)的减少烟丝“干头” 量的方法. 该方法利用生产实时数据作为输入特征向量感知烘丝生产过程的状态变化, 以烟丝含水率检测值为依据来评价、优化烘丝温度控制策略, 实现对烘丝机温度设定值的在线修正, 优化烘丝开始阶段的温度控制, 有效改善烟丝过干问题. 与烘丝机的自动控制模式和人工干预模式相比, 烟丝含水率的标准偏差比自动控制时降低了44.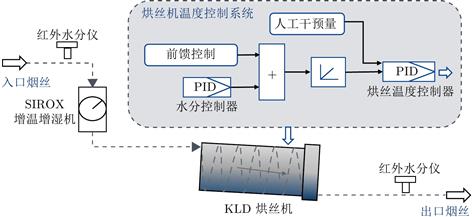 7%, 比人工干预时降低了14.3%. 实验结果表明烟丝含水率的稳定性有较大提高, 烟丝“干头” 量明显减少, 验证了所提方法的有效性和可行性.

|
关 键 词: | 烟丝含水率 过干烟丝 强化学习 超调 |
收稿时间: | 2019-05-14 |
|
| 点击此处可从《自动化学报》浏览原始摘要信息 |
|
点击此处可从《自动化学报》下载免费的PDF全文 |
|