全天实时跟踪无人机目标的多正则化相关滤波算法 |
| |
引用本文: | 王法胜, 李富, 尹双双, 王星, 孙福明, 朱兵. 全天实时跟踪无人机目标的多正则化相关滤波算法. 自动化学报, 2023, 49(11): 2409−2425 doi: 10.16383/j.aas.c220424 |
| |
作者姓名: | 王法胜 李富 尹双双 王星 孙福明 朱兵 |
| |
作者单位: | 1.大连民族大学信息与通信工程学院 大连 116600;2.哈尔滨工业大学电子与信息工程学院 哈尔滨 150001 |
| |
基金项目: | 国家自然科学基金(61972068,61976042);;国家重点研发计划(2021YFC3320300);;兴辽英才计划(XLYC2007023); |
| |
摘 要: | 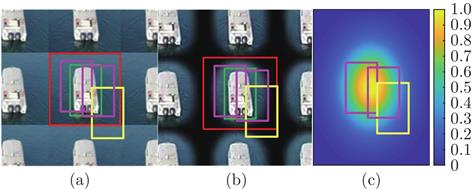 相关滤波算法(Correlation filter, CF)已广泛应用于无人机目标跟踪. 然而, 受无人机 (Unmanned aerial vehicle, UAV) 平台本身计算性能的制约, 现有的无人机相关滤波跟踪算法大都仅采用手工特征来描述目标的外观, 难以获得目标的全面语义信息. 并且这些跟踪算法仅能较好地进行光照条件良好场景下的跟踪, 而在跟踪夜间场景下的目标时性能严重下降. 此外, 相关滤波跟踪器采用余弦窗口来抑制循环移位产生的边界效应, 缩小了样本提取区域, 产生了训练样本污染的问题, 这不可避免地降低了跟踪器的性能. 针对以上问题, 提出全天实时多正则化相关滤波算法(All-day and real-time multi-regularized correlation filter, AMRCF)跟踪无人机目标. 首先, 引入一个自适应图像增强模块, 在不影响图像各通道颜色比例的前提下, 对获得的图像进行增强, 以提高夜间目标跟踪性能. 其次, 引入一个轻量型的深度网络来提取目标的深度特征, 并与手工特征一起来表示目标的语义信息. 此外, 在算法框架中嵌入高斯形状掩膜, 在抑制边界效应的同时, 有效避免训练样本污染. 最后, 在5个公开的无人机基准数据集上进行充分的实验. 实验结果表明, 所提出的算法与多个先进的相关滤波跟踪器相比, 取得了有竞争力的结果, 且算法的实时速度约为25 fps, 能够胜任无人机的目标跟踪任务.
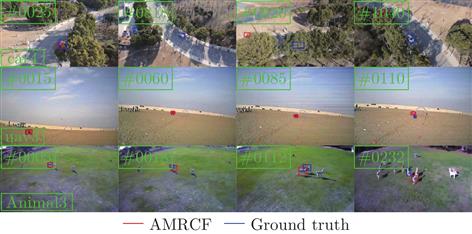
|
关 键 词: | 无人机目标跟踪 相关滤波 自适应图像增强模块 轻量型深度网络 高斯形状掩膜 |
收稿时间: | 2022-05-23 |
|
| 点击此处可从《自动化学报》浏览原始摘要信息 |
|
点击此处可从《自动化学报》下载免费的PDF全文 |
|