基于总体经验模态分解的多类特征的运动想象脑电识别方法研究 |
| |
引用本文: | 杨默涵, 陈万忠, 李明阳. 基于总体经验模态分解的多类特征的运动想象脑电识别方法研究. 自动化学报, 2017, 43(5): 743-752. doi: 10.16383/j.aas.2017.c160175 |
| |
作者姓名: | 杨默涵 陈万忠 李明阳 |
| |
作者单位: | 吉林大学通信工程学院分布式智能信息处理实验室 长春 130025 |
| |
基金项目: | 吉林省科技发展计划自然基金(20150101191JC),吉林大学研究生创新基金(2016092)资助 |
| |
摘 要: | 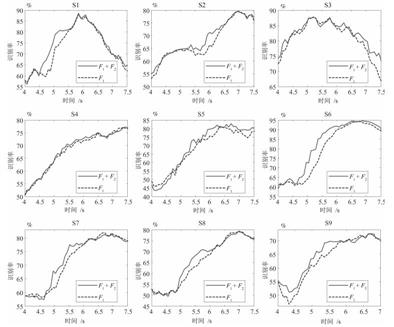 人的脑电信号(Electroencephalogram,EEG)复杂且具有非线性及非平稳性的特点使其不易分析处理,其识别效果也依赖于数据集的不同,而表现不稳定.本文中应用的总体经验模态分解(Ensemble empirical mode decomposition,EEMD)是一种具有强自适应性的信号处理方法,其在时频域展现的良好分辨率特别适合脑电识别任务处理.本文提出利用EEMD分解后得到的较具影响能力的固有模态函数(Intrinsic mode functions,IMFs),利用希尔伯特变换提取边际谱(Marginal spectrum,MS)及瞬时能谱(Instantaneous energy spectrum,IES)时频特征,同时通过加窗的方法提取非线性动力学特征近似熵特征,利用线性判别分类器(Linear discriminant analysis,LDA)作为分类器,实验结果得出,对于被试S2和被试S3可达到识别率分别为79.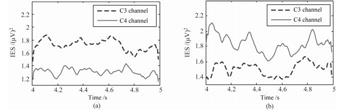 60%和87.77%,实验中9名被试的平均识别率为82.74%,得到平均识别率也高于近期使用相同数据集文献的其他方法.
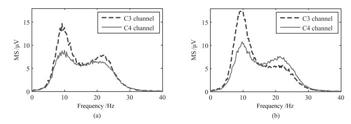
|
关 键 词: | 脑电信号 运动想象 总体经验模态分解 线性判别分类器 |
收稿时间: | 2016-03-03 |
|
| 点击此处可从《自动化学报》浏览原始摘要信息 |
|
点击此处可从《自动化学报》下载免费的PDF全文 |
|