基于改进注意力机制的探地雷达图像反演 |
| |
引用本文: | 李春堂,李旭. 基于改进注意力机制的探地雷达图像反演[J]. 电波科学学报,2023,38(5):825-834. DOI: 10.12265/j.cjors.2022175 |
| |
作者姓名: | 李春堂 李旭 |
| |
作者单位: | 1.东南大学软件学院, 南京 210096;2.东南大学仪器科学与工程学院, 南京 210096 |
| |
基金项目: | 国家重点研发计划(2019YFC1511505);国家自然科学基金(61973079);江苏省重点研发计划(BE2022053-5) |
| |
摘 要: | 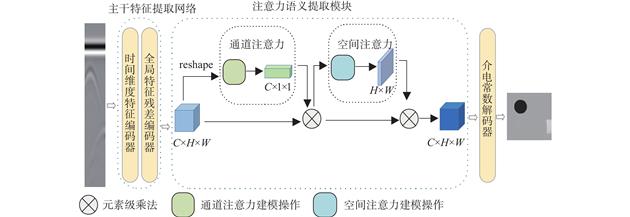 探地雷达(ground penetrating radar,GPR)是一种重要的无损检测工具,被广泛应用于道路缺陷检测、路面以下物体检测以及非结构化地形感知等领域. 针对基于深度学习的介电常数图反演方法特征提取不全、准确度不高的局限性,本文引入注意力机制, 提出了一种GPR图像智能反演算法,设计了适用于GPR图像反演的神经网络InvNet以完成从GPR图像到介电常数图的非线性映射. 该算法首先利用时间维度卷积和全局特征残差编码器提取GPR时间维度和全局特征,接着采用改进的注意力机制作为注意力语义提取模块提取特征图的全局语义信息,最终通过介电常数解码器完成GPR介电常数图重构. 在基于时域有限差分法构建的GPR反演数据集上,InvNet反演结果的结构相似性指数(structural similarity index measure,SSIM)达到了97.14%,均方误差(mean square error, MSE)降为0.003 0,平均绝对误差(mean absolute error,MAE)降为0.015 7;相比于最新GPR反演网络算法GPRInvNet和PINet,InvNet的SSIM分别提高了1.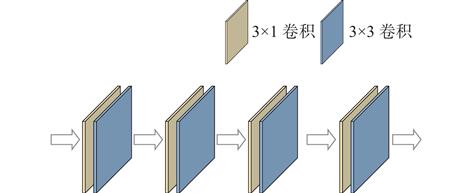 06%和0.86%,MSE分别降低了0.24%和0.25%,MAE分别降低了1.41%和1.02%. 实验表明本文方法能够根据GPR图像有效反演出对应的介电常数模型图,并且与现有方法的对比结果展示了其优越的反演性能.

|
关 键 词: | 探地雷达 无损检测 图像反演 深度学习 注意力机制 |
收稿时间: | 2022-08-23 |
|
| 点击此处可从《电波科学学报》浏览原始摘要信息 |
|
点击此处可从《电波科学学报》下载免费的PDF全文 |
|