一种结合三重注意力机制的双路径网络胸片疾病分类方法 |
| |
引用本文: | 李锵, 王旭, 关欣. 一种结合三重注意力机制的双路径网络胸片疾病分类方法[J]. 电子与信息学报, 2023, 45(4): 1412-1425. doi: 10.11999/JEIT220172 |
| |
作者姓名: | 李锵 王旭 关欣 |
| |
作者单位: | 天津大学微电子学院 天津 300072 |
| |
基金项目: | 国家自然科学基金(61471263, 61872267, 62071323),天津市自然科学基金(16JCZDJC31100),天津市科技计划项目(20YDTPJC01110),天津大学自主创新基金(2021XZC-0024) |
| |
摘 要: |  近年来,利用CNN进行医学图像处理,在胸片疾病分类任务中取得显著研究进展。然而,与单一结构CNN相比,双路径网络可结合不同CNN特点,从而提高疾病分类能力。其次,对于不同疾病,其位置、大小、形态、密度、纹理等特征均有不同,而注意力机制有助于模型提取不同病理特征,提升分类精度。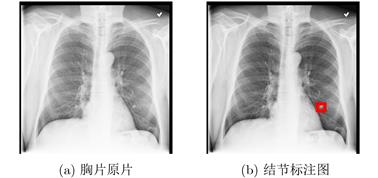 因此针对胸片疾病分类问题,该文提出一种结合三重注意力机制的双路径卷积神经网络(TADPN),TADPN将ResNet和DenseNet结合的双路径网络DPN作为骨干网络,并利用3种不同形式的注意力机制改进DPN,在维持参数量稳定的同时提高网络复杂度,进而提升对胸片疾病的分类精度。在ChestXray14数据集上实验,并与目前较为先进的6种算法对比,14种疾病的平均AUC值达到0.8185,较前人提升1.1%,表明双路径CNN及三重注意力机制对胸片疾病分类的有效性及TADPN的先进性。

|
关 键 词: | 医学图像处理 胸片分类 卷积神经网络 注意力机制 |
收稿时间: | 2022-02-22 |
修稿时间: | 2022-07-27 |
|
| 点击此处可从《电子与信息学报》浏览原始摘要信息 |
|
点击此处可从《电子与信息学报》下载免费的PDF全文 |
|